Artificial intelligence (AI) is revolutionizing material discovery by enabling researchers to find new magnetic properties that can lead to electronics without energy loss. A team of scientists from MIT, Pennsylvania State University, and the National Institute of Standards and Technology has developed a novel method that combines polarized neutron reflectometry (PNR) with neural networks to detect an intriguing phenomenon called the magnetic proximity effect.
What is the magnetic proximity effect?
The magnetic proximity effect occurs when magnetism penetrates slightly into the surface of a topological material that is normally nonmagnetic. Topological materials are a new class of quantum materials that offer robustness against disturbances and potential applications in dissipationless electronics. The magnetic proximity effect can induce dissipationless electronic states on the surface of topological materials, but it is very subtle and hard to observe with traditional methods.
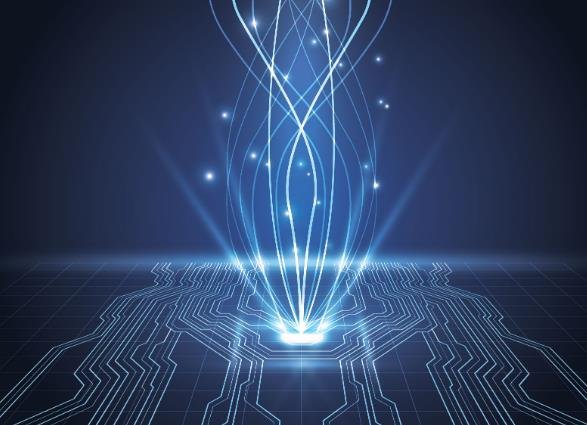
How does AI help?
The researchers used AI to enhance their analysis of PNR data, which measures the depth-dependent magnetic structure of multilayered materials. PNR works by reflecting two polarized neutron beams with opposing spins from the sample and collecting them on a detector. If the neutron encounters a magnetic flux that has the opposite orientation, it will change its spin state, resulting in different signals measured from the spin up and spin down neutron beams.
However, interpreting PNR data can be challenging due to noise and ambiguity. The researchers trained a neural network to learn from simulated PNR data and then applied it to real experimental data. The neural network was able to extract the magnetic profile of the sample with high accuracy and sensitivity, revealing the presence of the magnetic proximity effect.
What are the implications?
The researchers demonstrated their method on a multilayered material composed of chromium triiodide (CrI3), a magnetic material, and tungsten diselenide (WSe2), a topological material. They found that the magnetic proximity effect extended about 1 nanometer into the WSe2 layer, which is consistent with theoretical predictions. They also discovered that the magnetic proximity effect was tunable by applying an external electric field, which could open up new possibilities for controlling the electronic properties of topological materials.
The researchers believe that their method can be generalized to other types of materials and experiments, such as X-ray reflectometry and scanning tunneling microscopy. They hope that their work will inspire more collaborations between material scientists and AI experts to accelerate the discovery of new materials and phenomena.